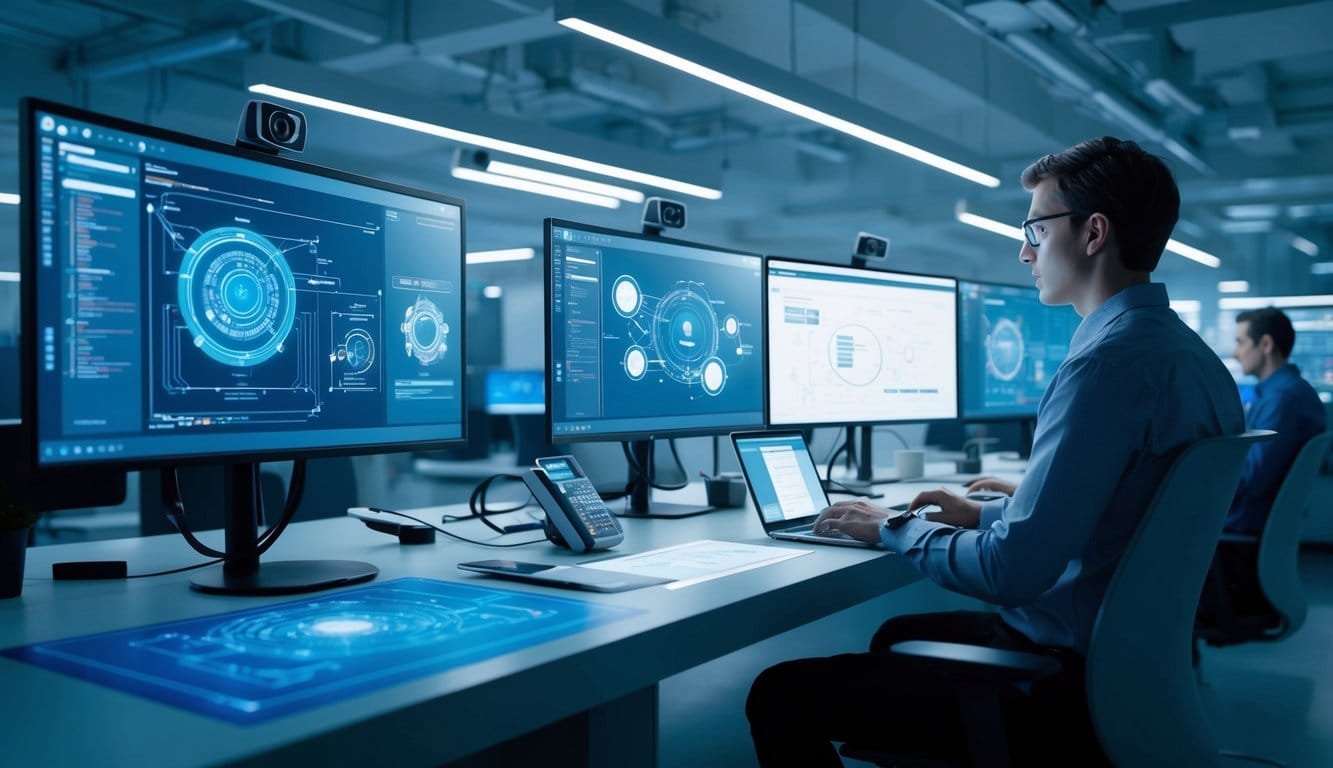
A revolutionary leap in artificial intelligence is poised to redefine engineering problem-solving, speeding up the resolution of complex analytical challenges to an unprecedented degree. With the advent of this cutting-edge AI system, personal computers can execute intricate mathematical computations at rates that surpass even the most powerful supercomputers, fundamentally altering how engineers model scenarios such as car crash impacts, spacecraft durability, and bridge stress resistance—potentially enhancing processing speeds by a staggering factor of thousands.
Introducing DIMON
The transformative framework, known as DIMON (Diffeomorphic Mapping Operator Learning), emerges as a robust tool capable of swiftly delivering solutions to critical mathematical equations essential for simulating phenomena like fluid dynamics and electrical currents across diverse geometric structures. These simulations are vital for standard assessments within the engineering world. Researchers have shared their findings in the journal Nature Computational Science.
DIMON particularly excels in addressing partial differential equations (PDEs), which are foundational to scientific and engineering research. These equations allow researchers to model the evolution of physical systems over time and space. The implications of this innovation are vast and far-reaching, with applications across multiple engineering disciplines that include, but are not limited to, crash testing and health diagnostics.
Applications in Cardiac Research
To showcase DIMON’s prowess, the research team undertook an ambitious project involving over 1,000 digital twin models of human hearts. These meticulously crafted computer representations of real patients’ anatomies served as the testing ground for the AI framework. Remarkably, DIMON exhibited impressive accuracy in forecasting the propagation of electrical signals through these uniquely shaped hearts.
The examination focused on partial differential equations to deepen the understanding of cardiac arrhythmias—aberrant electrical impulses that throw the heart’s rhythm into chaos. By utilizing these digital twins, researchers not only assess risks associated with potentially life-threatening arrhythmias but also devise tailored treatment strategies that could save lives.
Transforming Computational Efficiency
The advent of this novel AI technology promises to upend persistent challenges that have long plagued advanced medical technologies. In the past, obtaining results after scanning a patient’s heart would take about a week. Yet, with this innovative AI approach, researchers anticipate a dramatic compression of that timeframe, reducing the calculations from several hours to just 30 seconds. This leap makes it feasible to conduct these evaluations using everyday desktop computers, seamlessly integrating advanced technology into routine clinical practice.
Traditionally, solving partial differential equations involves breaking down complex shapes—be it aircraft wings or the intricate features of human organs—into smaller grids or meshes. Each segment is tackled separately before being recombined, a process that becomes increasingly resource-intensive when shapes undergo changes due to impact or deformation.
DIMON dramatically streamlines this process by employing AI to predict system behavior across various geometric configurations, eliminating the need for exhaustive recalculations every time a modification occurs. By identifying patterns in essential physical variables such as thermal dynamics, stress, and motion, DIMON enhances computation efficiency, proving particularly advantageous for design optimizations and tailored modeling scenarios.
The framework also holds promise for advancing the understanding of cardiac conditions linked to arrhythmias. Its adaptability positions DIMON as a versatile solution for diverse engineering challenges that repeatedly require resolving partial differential equations across varying shapes. Researchers express enthusiasm for exploring DIMON’s capabilities, eager to share its potential with the broader community and foster innovations in engineering design solutions.
“`htmlStudy Details:
- Title: A scalable framework for learning the geometry-dependent solution operators of partial differential equations
- Authors: Minglang Yin, Nicolas Charon, Ryan Brody, Lu Lu, Natalia Trayanova, Mauro Maggioni
- Journal: Nature Computational Science
- Publication Date: 2024
- DOI: 10.1038/s43588-024-00732-2